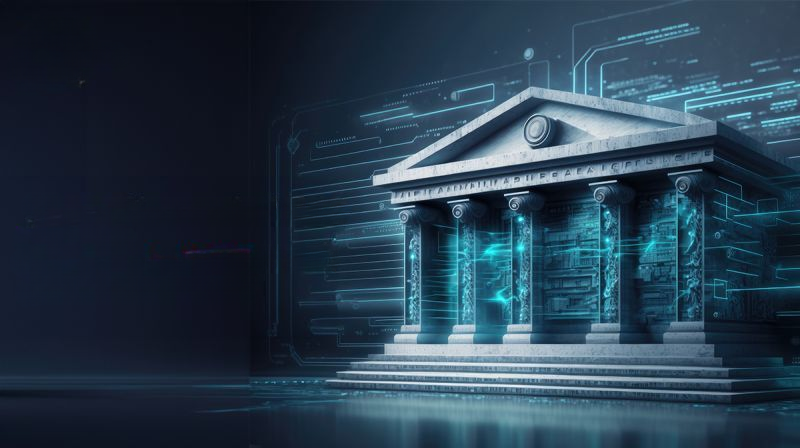
Machine Learning in Credit Risk Assessment: Transforming Banking Practices
In the dynamic world of finance, where lending and credit play a pivotal role, the accuracy of credit risk assessment is paramount for the stability of banks and financial institutions. Traditional credit risk evaluation methods have their limitations, often relying heavily on historical data and fixed models. In contrast, machine learning has emerged as a game-changer, revolutionizing how banks approach credit risk assessment. This article explores the integration of machine learning techniques in credit risk evaluation, highlighting its benefits, challenges, and the way forward.
The Evolution of Credit Risk Assessment:
Historically, banks have employed rule-based approaches and statistical models to assess credit risk. These methods, while effective to some extent, may fall short when dealing with complex, ever-changing financial landscapes. Machine learning, a subset of artificial intelligence, offers a data-driven approach that can analyze vast volumes of data, identify patterns, and make predictions that were previously unattainable.
Benefits of Machine Learning in Credit Risk Assessment:
- Enhanced Accuracy: Machine learning algorithms can process a wide range of data sources, including unconventional ones like social media activity, to develop a more comprehensive and accurate credit risk profile of an individual or business.
- Dynamic Modeling: Unlike fixed statistical models, machine learning algorithms can adapt to changing economic conditions and evolving borrower behavior, ensuring more timely and relevant risk assessments.
- Predictive Power: Machine learning can predict potential defaulters more effectively by identifying subtle trends and correlations that might be missed by traditional methods.
- Personalized Risk Evaluation: Machine learning enables banks to offer tailored credit solutions based on individual risk profiles, leading to better customer experiences and improved loan performance.
- Automation and Efficiency: Automated data analysis and decision-making streamline the credit evaluation process, reducing the time and resources required for loan approval.
Challenges and Considerations:
- Data Quality and Bias: Machine learning models heavily rely on data quality. Incomplete or biased data can lead to skewed predictions, emphasizing the importance of robust data governance and preprocessing.
- Interpretability: Some advanced machine learning models, such as neural networks, can be difficult to interpret. Banks must strike a balance between model complexity and the need for understandable outcomes.
- Regulatory Compliance: Adhering to regulations and ensuring the transparency of automated credit decisions is crucial to maintaining trust and regulatory compliance.
Implementation and Future Outlook:
To successfully implement machine learning in credit risk assessment, banks must:
- Data Infrastructure: Develop a robust data infrastructure that can efficiently gather, store, and preprocess diverse data sources.
- Model Selection: Choose appropriate machine learning algorithms based on the specific credit risk assessment needs and data available.
- Monitoring and Validation: Continuously monitor and validate machine learning models to ensure their accuracy and consistency over time.
- Collaboration: Collaborate with regulators, industry experts, and technology partners to ensure alignment with best practices and regulatory requirements.
The integration of machine learning in credit risk assessment is already underway in progressive financial institutions. As technology continues to advance, we can expect even more sophisticated models that can handle complex financial scenarios and adapt to rapid changes in the market.
Conclusion:
Machine learning’s ability to process and analyze vast amounts of data has the potential to transform credit risk assessment in banking. By leveraging these advanced techniques, banks can make more accurate predictions, offer personalized financial solutions, and ultimately enhance their ability to manage credit risk effectively. As banks embrace this paradigm shift, they must remain vigilant in addressing challenges and collaborate with industry stakeholders to ensure a responsible and seamless transition to a new era of credit risk assessment.